ABOUT
Deep learning to design imaging hardware
This page is dedicated to deep learning algorithms that design new types of hardware. The primary focus of the work presented here is to showcase new and improved imaging systems (cameras, microscopes, CT, MRI), which are specifically optimized to collect data by and for deep learning tasks. Please find an introduction to this area of research here, and several example projects demonstrating this new effort below.
Duke Computational Optics Lab
This website includes research from the Computational Optics Lab at Duke University. We develop new microscopes, cameras and computer algorithms to capture better biomedical images. The lab is directed by Dr. Roarke Horstmeyer , who is an Assistant Professor in the Department of Biomedical Engineering at Duke
BME Course: Machine Learning and Imaging
The Duke University engineering course, Machine Learning and Imaging, is now fully online. This class is an overview of machine learning and imaging science, with a focus on the intersection of the two fields. It offers much of the introductory material needed to understand the basics of machine learning for hardware design. Please find lectures, homeworks, example code and the course content here
Projects
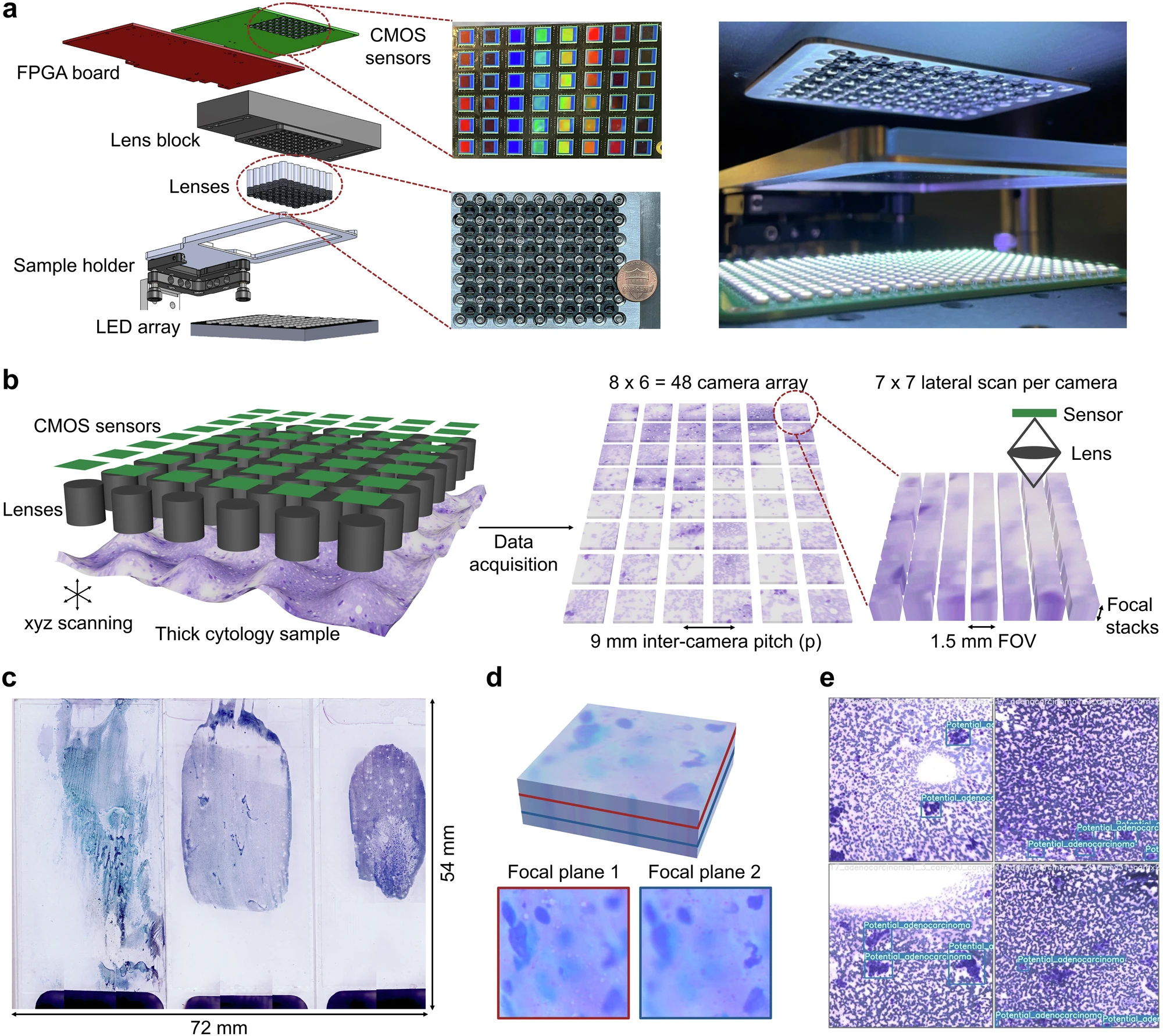
Rapid 3D imaging at cellular resolution for digital cytopathology with a multi-camera array scanner (MCAS)
Optical microscopy has long been the standard method for diagnosis in cytopathology. Whole slide scanners can image and digitize large sample areas automatically, but are slow, expensive and therefore not widely available. Clinical diagnosis of cytology specimens is especially challenging since these samples are both spread over large areas and thick, which requires 3D capture. Here, we introduce a new parallelized microscope for scanning thick specimens across extremely wide fields-of-view (54×72 mm2) at 1.2 and 0.6 µm resolutions, accompanied by machine learning software to rapidly assess these 16 gigapixel scans. This Multi-Camera Array Scanner (MCAS) comprises 48 micro-cameras closely arranged to simultaneously image different areas. By capturing 624 megapixels per snapshot, the MCAS is significantly faster than most conventional whole slide scanners. We used this system to digitize entire cytology samples (scanning three entire slides in 3D in just several minutes) and demonstrate two machine learning techniques to assist pathologists: first, an adenocarcinoma detection model in lung specimens (0.73 recall); second, a slide-level classification model of lung smears (0.969 AUC).
npj Imaging (2024)
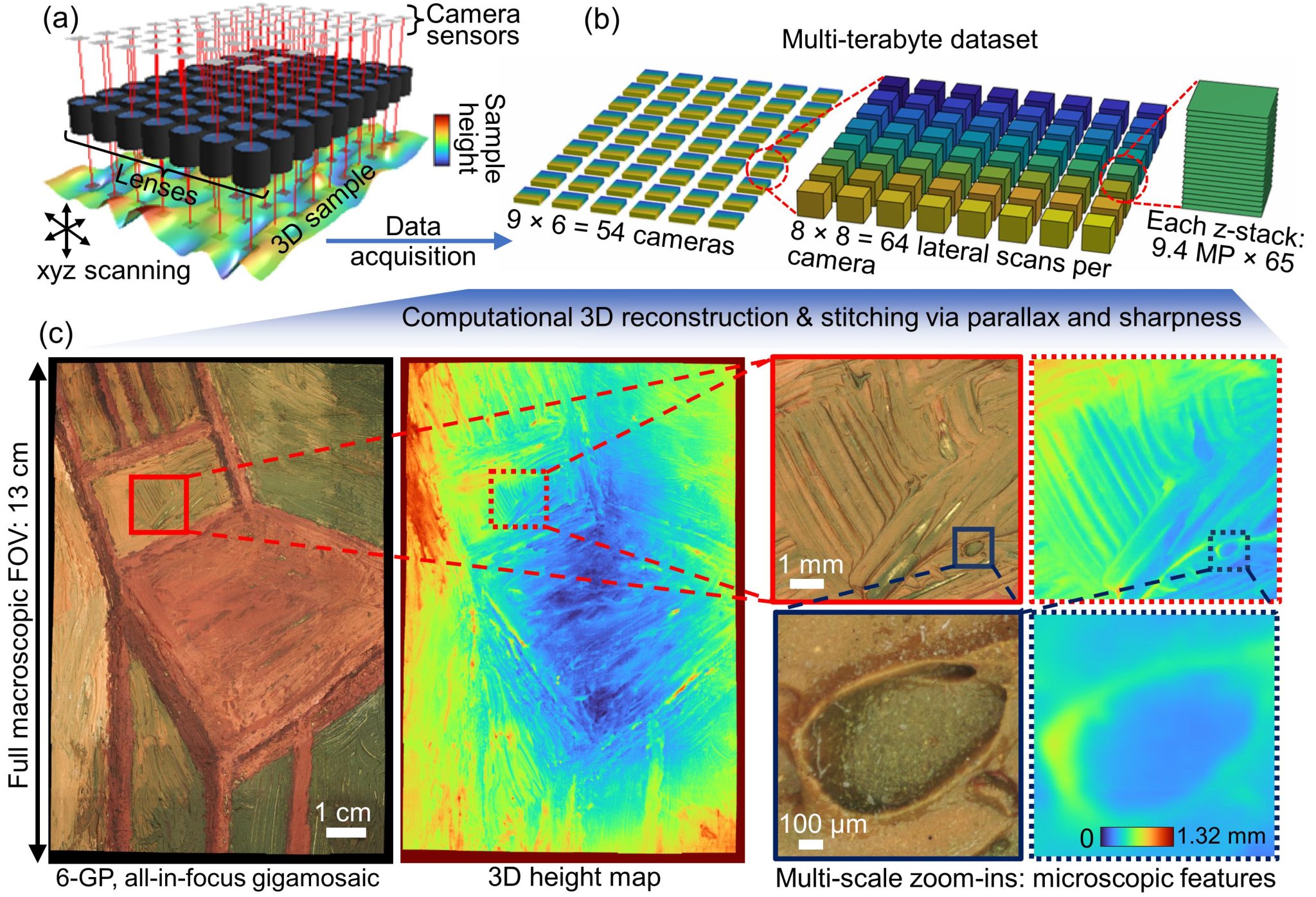
Computational 3D topographic microscopy from terabytes of data per sample
We present a large-scale computational 3D topographic microscope that enables 6-gigapixel profilometric 3D imaging at micron-scale resolution across >110 cm2 areas over multi-millimeter axial ranges. Our computational microscope, termed STARCAM (Scanning Topographic All-in-focus Reconstruction with a Computational Array Microscope), features a parallelized, 54-camera architecture with 3-axis translation to capture, for each sample of interest, a multi-dimensional, 2.1-terabyte (TB) dataset, consisting of a total of 224,640 9.4-megapixel images. We developed a self-supervised neural network-based algorithm for 3D reconstruction and stitching that jointly estimates an all-in-focus photometric composite and 3D height map across the entire field of view, using multi-view stereo information and image sharpness as a focal metric. The memory-efficient, compressed differentiable representation offered by the neural network effectively enables joint participation of the entire multi-TB dataset during the reconstruction process. To demonstrate the broad utility of our new computational microscope, we applied STARCAM to a variety of decimeter-scale objects, with applications ranging from cultural heritage to industrial inspection.
Journal of Big Data (2024)
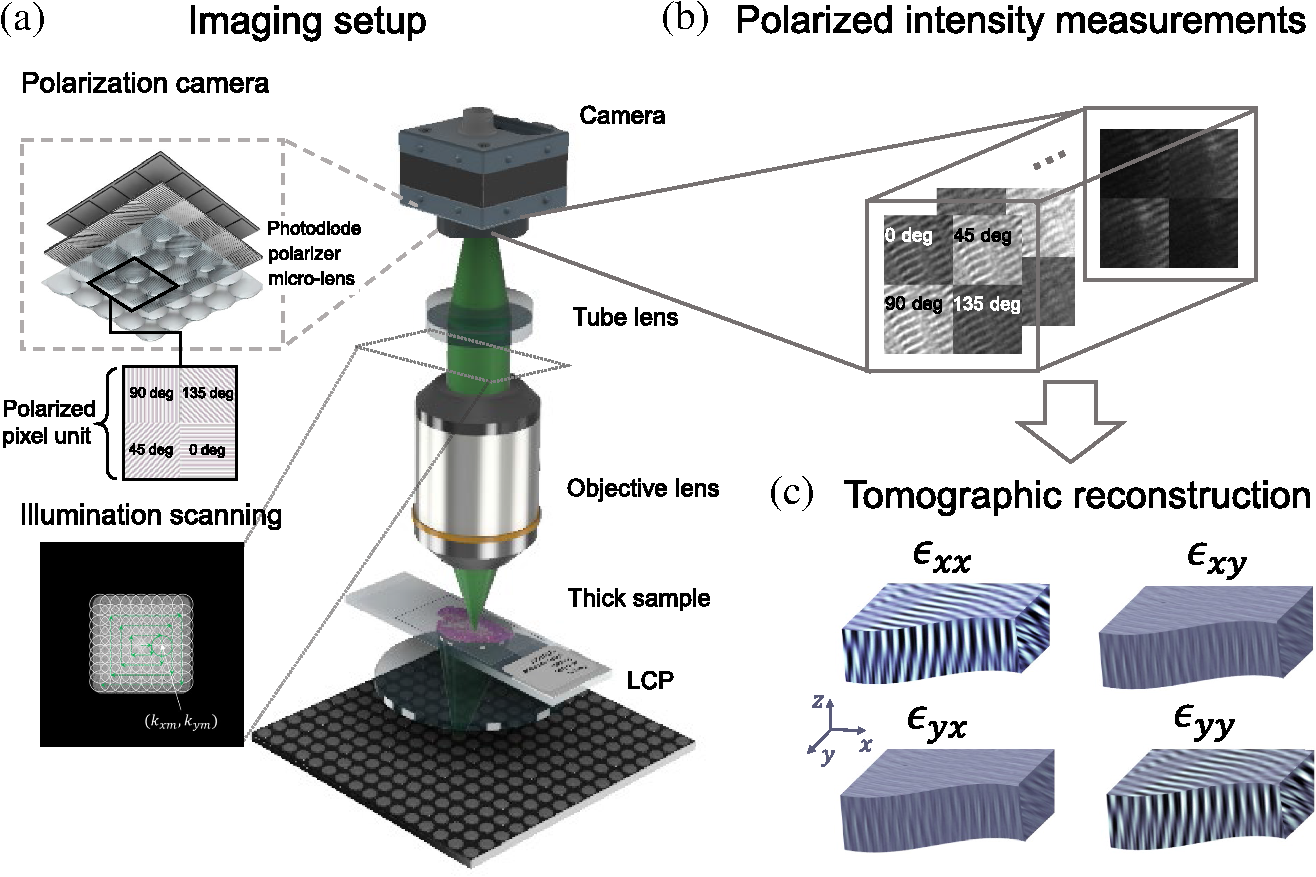
T2oFu: Tensorial tomographic Fourier ptychography with applications to muscle tissue imaging
We report tensorial tomographic Fourier ptychography (T2oFu), a nonscanning label-free tomographic microscopy method for simultaneous imaging of quantitative phase and anisotropic specimen information in 3D. Built upon Fourier ptychography, a quantitative phase imaging technique, T2oFu additionally highlights the vectorial nature of light. We demonstrate T2oFu’s efficiency through volumetric reconstructions of refractive index, birefringence, and orientation for various validation samples, as well as tissue samples from muscle fibers and diseased heart tissue.
Advanced Photonics (2024)
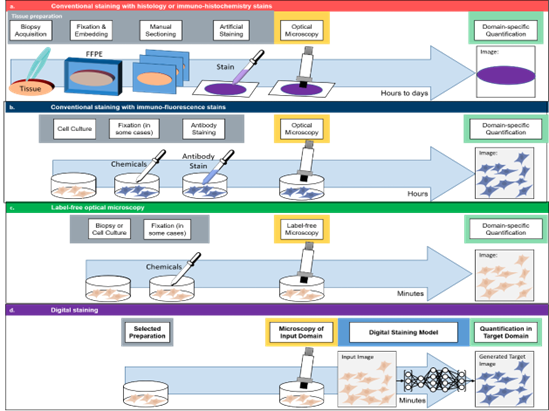
Our review on Digital staining in optical microscopy using deep learning
Over the past few years, digital staining has emerged as a promising concept to use modern deep learning for the translation from optical contrast to established biochemical contrast of actual stainings. In this review article, we provide an in-depth analysis of the current state-of-the-art in this field, suggest methods of good practice, identify pitfalls and challenges and postulate promising advances towards potential future implementations and applications.
PhotoniX (2023)
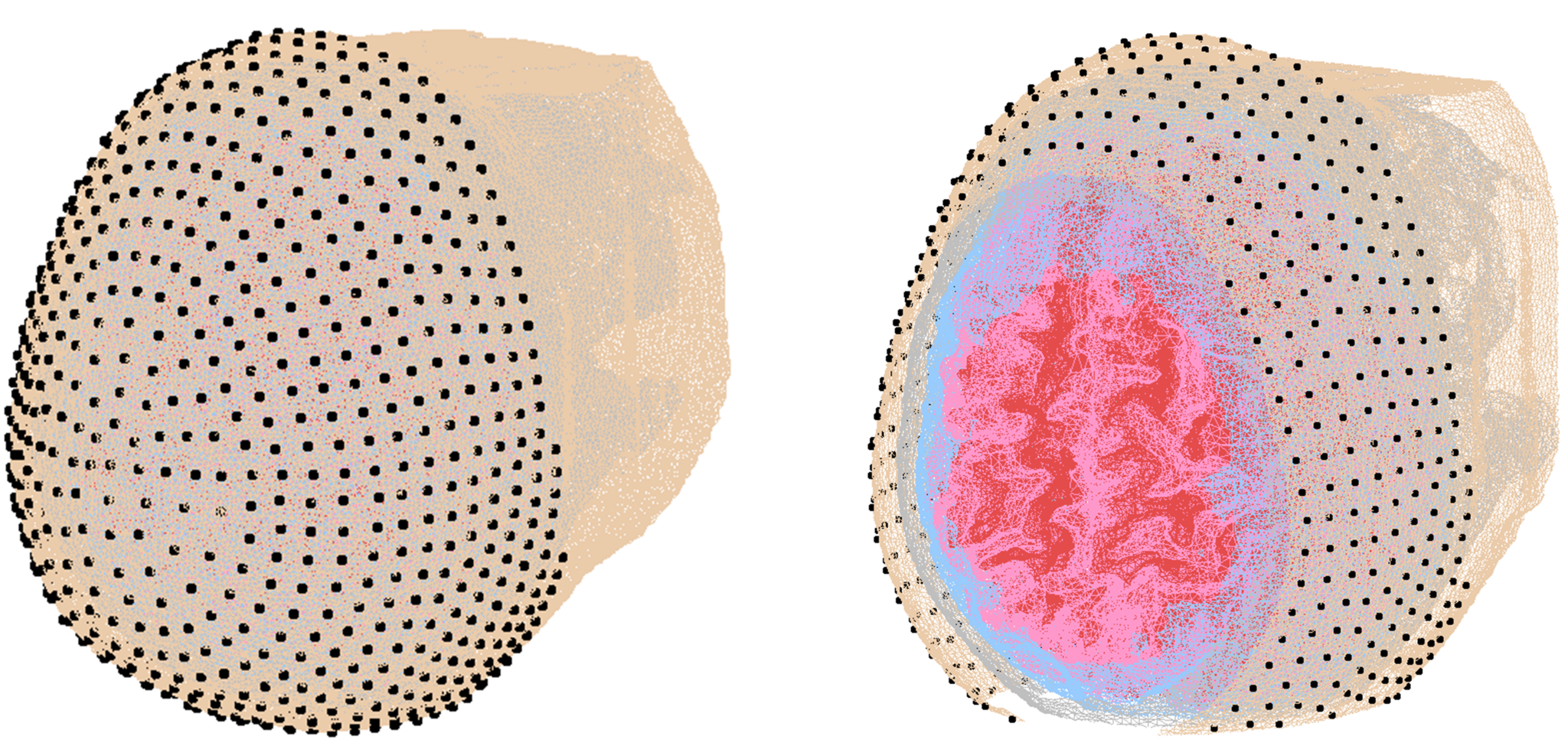
scatterBrains: an open database of human head models and companion optode locations for realistic Monte Carlo photon simulations
In this work, we provide the NIRS/DCS communities with an open-source, user-friendly database of morphologically and optically realistic head models, as well as a succinct software pipeline to prepare these models for mesh-based Monte Carlo simulations of light transport. The models, head surface locations, and all associated code are freely available under the scatterBrains project on Github. We hope this will make MRI-based head models and virtual optode placement easily accessible to all. Contributions to the database are welcome and encouraged.
Journal of Biomedical Optics (2023)
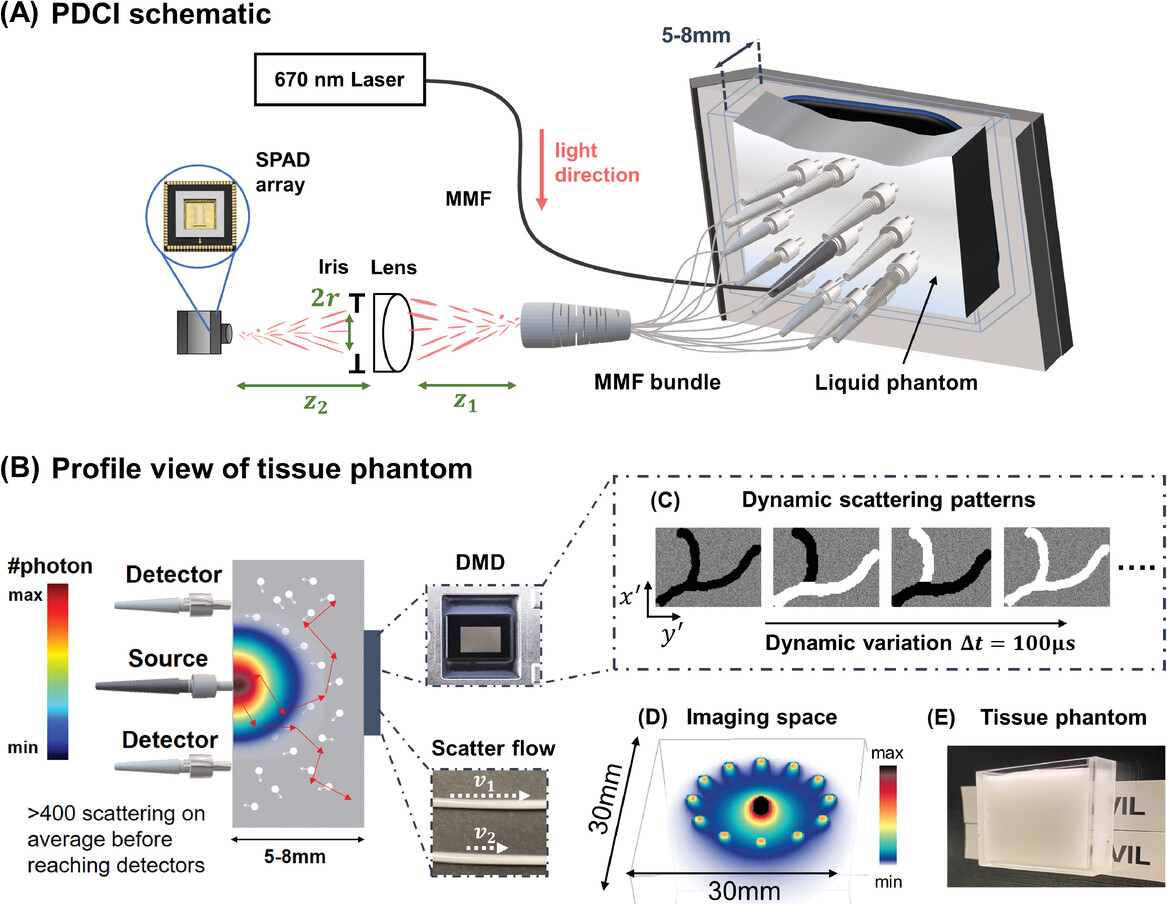
PDCI: Imaging Dynamics Beneath Turbid Media via Parallelized Single-Photon Detection
In this work, a single-photon avalanche diode array camera is utilized to simultaneously monitor the temporal dynamics of speckle fluctuations at the single-photon level from 12 different phantom tissue surface locations delivered via a customized fiber bundle array. Then a deep neural network is applied to convert the acquired single-photon measurements into video of scattering dynamics beneath rapidly decorrelating tissue phantoms. The ability to reconstruct images of transient (0.1–0.4 s) dynamic events occurring up to 8 mm beneath a decorrelating tissue phantom with millimeter-scale resolution is demonstrated, and it is highlighted how the model can flexibly extend to monitor flow speed within buried phantom vessels.
Advanced Science (2022)
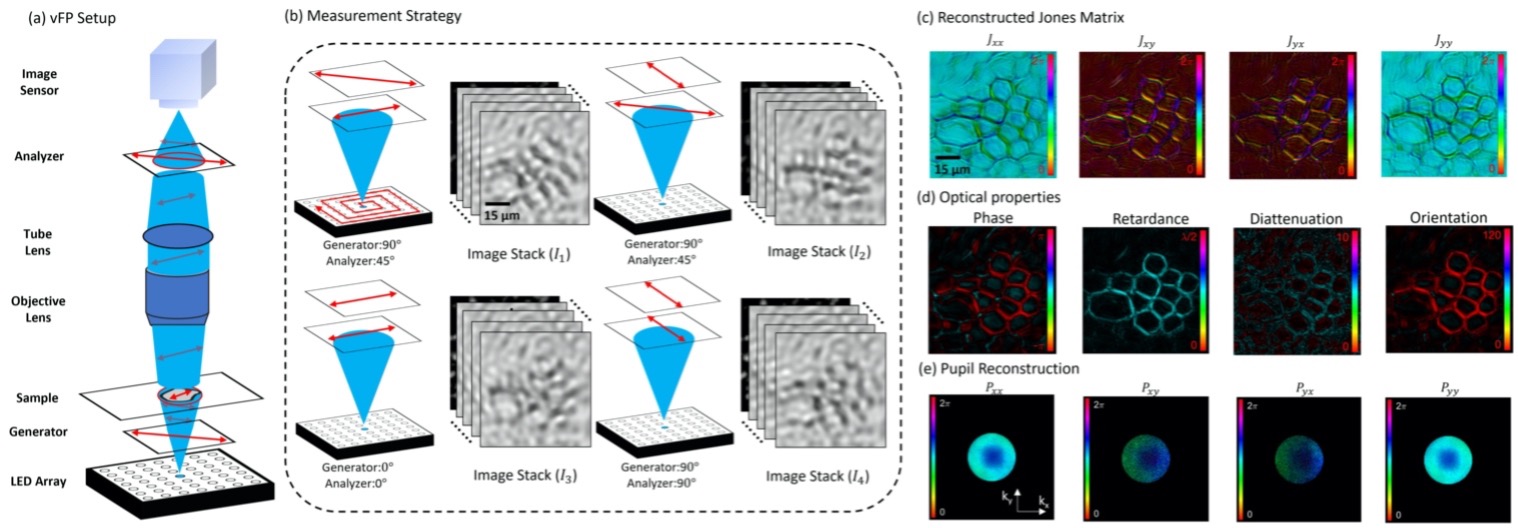
Vectorial FP
We present a microscopic imaging technique that uses variable-angle illumination to recover the complex polarimetric properties of a specimen at high resolution and over a large field-of-view. The approach extends Fourier ptychography, to additionally account for the vectorial nature of light.
Biomedical Optics Express (2022)
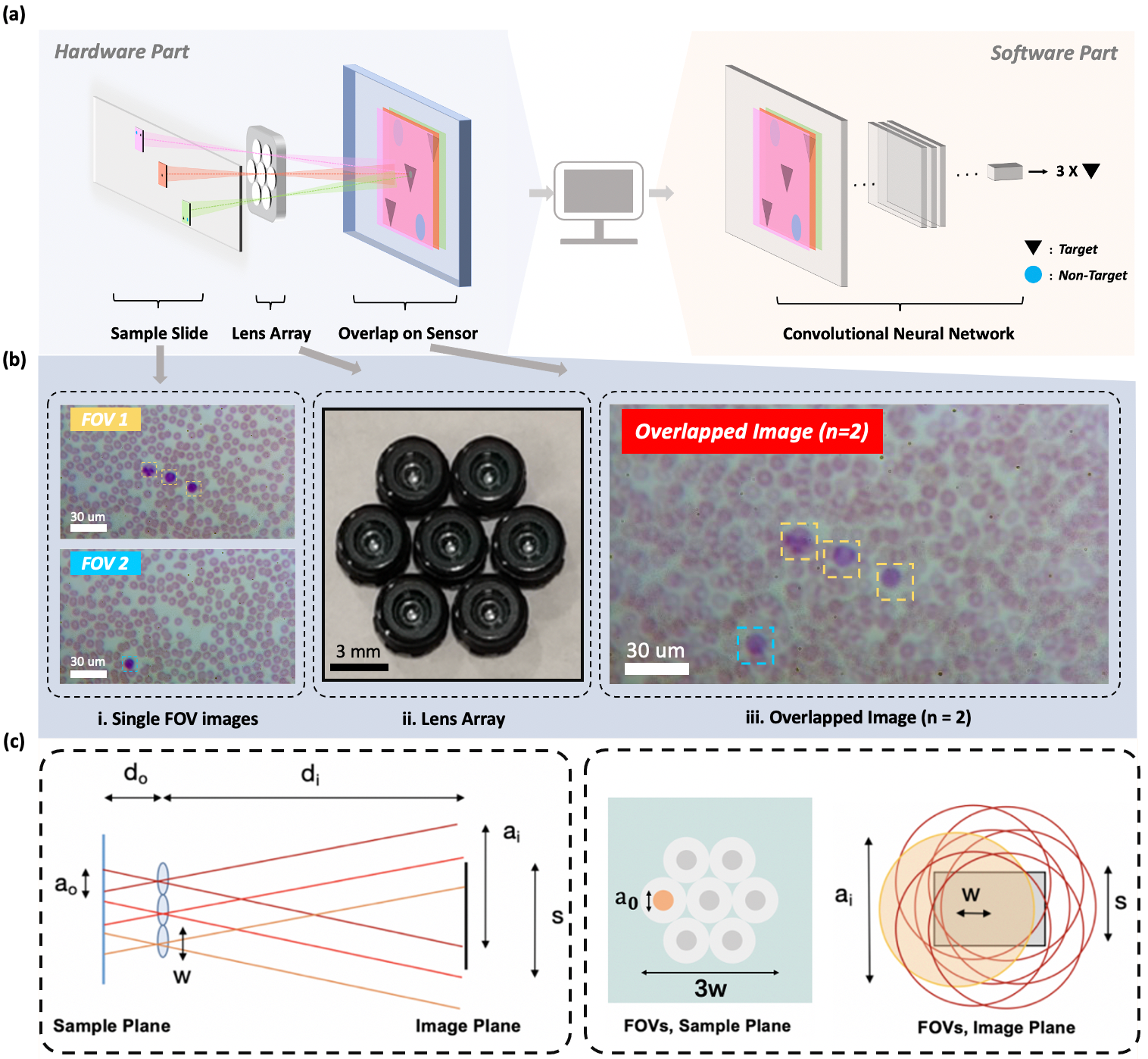
Overlapped Imaging
This work demonstrates a multi-lens microscopic imaging system that overlaps multiple independent fields of view on a single sensor for high-efficiency automated specimen analysis both in simulations and experiment. Our co-designed analysis software can achieve accurate detection of diagnostically-relevant features for several applications such malarial parasite detection and WBC counting.
Optics Express (2022)
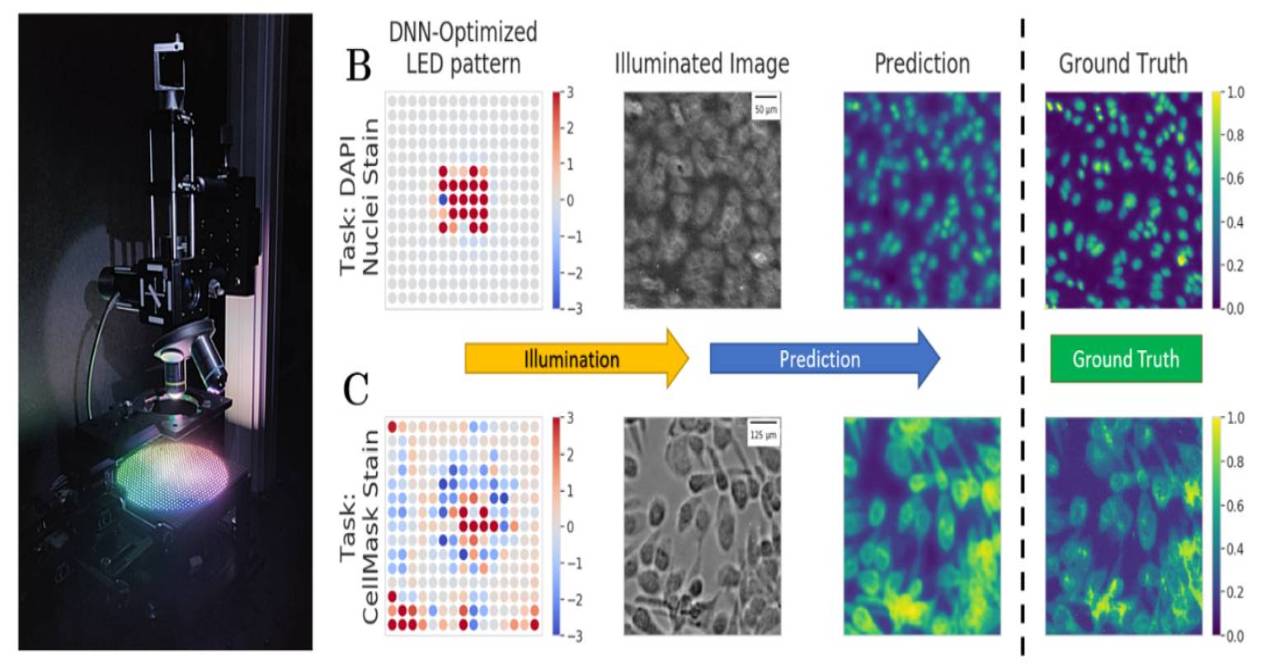
Virtual Fluoroscence
Our results show that by including a model of illumination within the first layers of a deep convolutional neural network, it is possible to learn task-specific LED patterns that substantially improve the ability to infer fluorescence image information from unstained transmission microscopy images.
ICCV (2021)
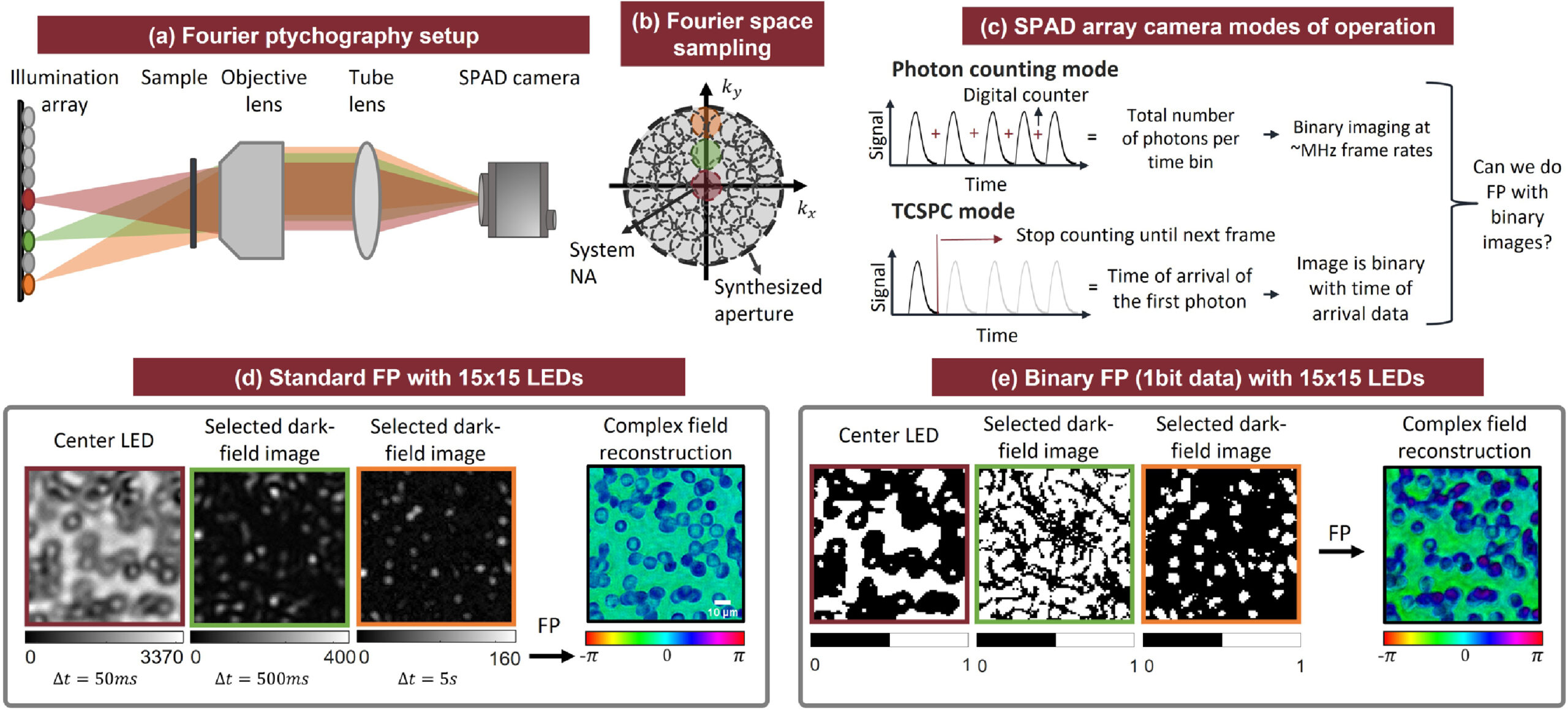
SPAD FP
We study the feasibility of implementing Fourier ptychography (FP) with SPAD array cameras to reconstruct an image with higher resolution and larger dynamic range from acquired binary intensity measurements.
Photonics Research (2021)
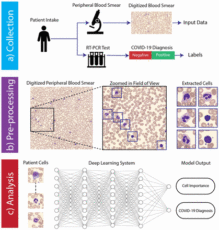
Deep Optical Blood Analysis
We propose a novel machine learning approach that offers a powerful and scalable means to analyse peripheral blood smears by utilizing a multiple instance learning based approach to understand the morphological impact of COVID-19 on the blood cells across the various cell types, which is still not well understood.
medRxiv (2021)
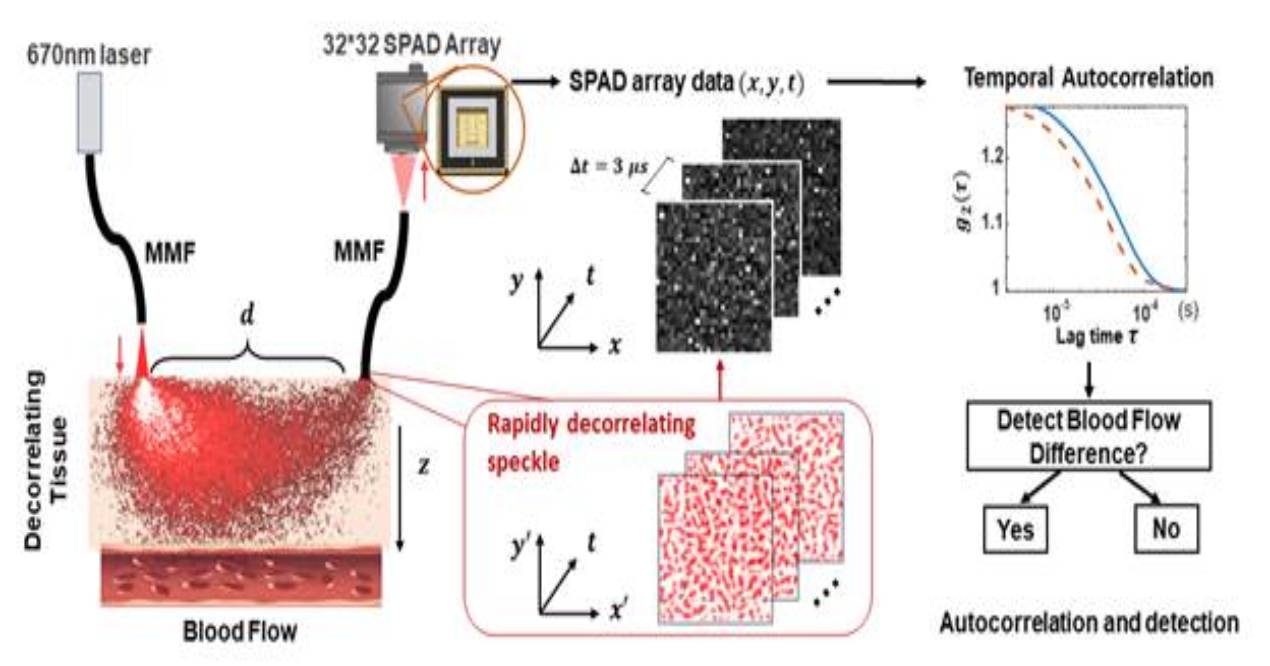
Parallelized Diffuse Correlation Spectrocopy
With a high-sensitivity DCS system with 1024 parallel detection channels integrated within a SPAD array, we demonstrate the ability to detect mm-scale perturbations up to 1 cm deep within a tissue-like phantom . We also measure the human pulse at high fidelity and detect behaviorally-induced physiological variations from the human prefrontal cortex.
APL Photonics (2020)
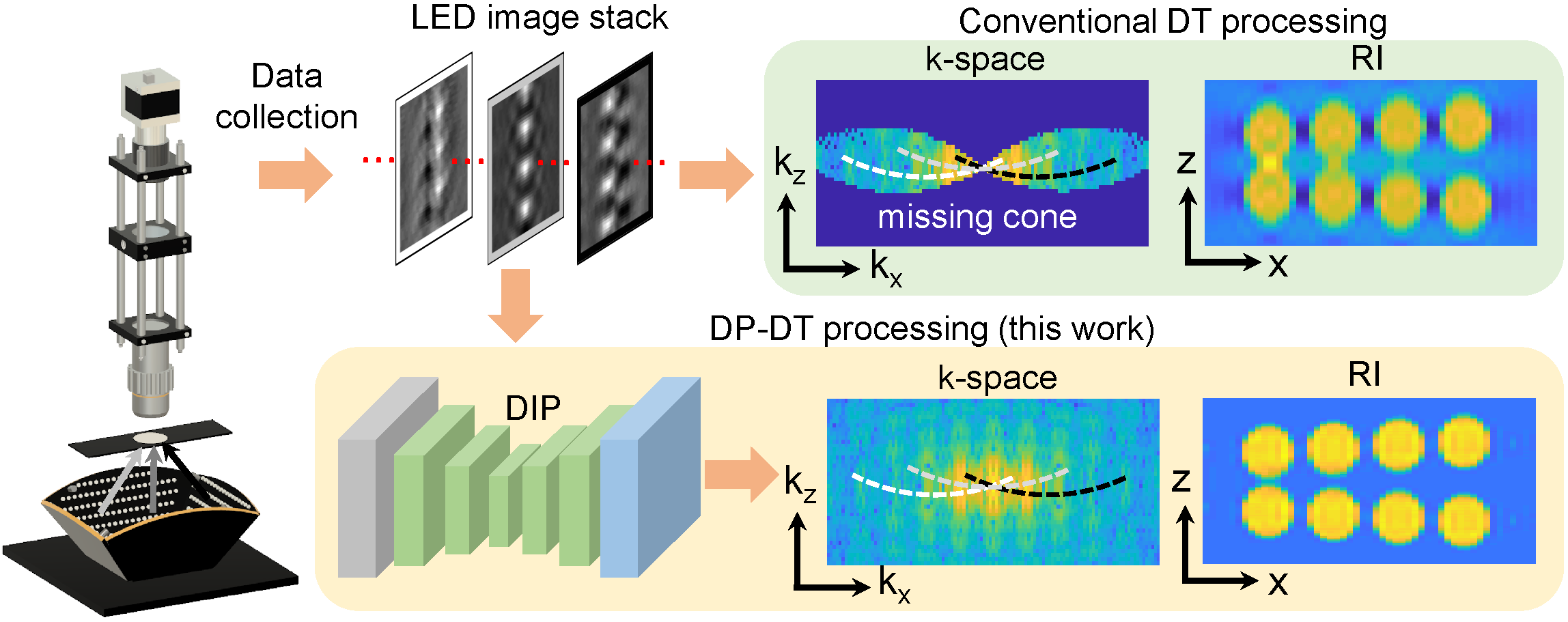
Deep Prior Diffraction Tomography
We present a tomographic imaging technique, termed Deep Prior Diffraction Tomography (DP-DT), to reconstruct the 3D refractive index (RI) of thick biological samples at high resolution from a sequence of low-resolution images collected under angularly varying illumination.
Optics Express (2020)
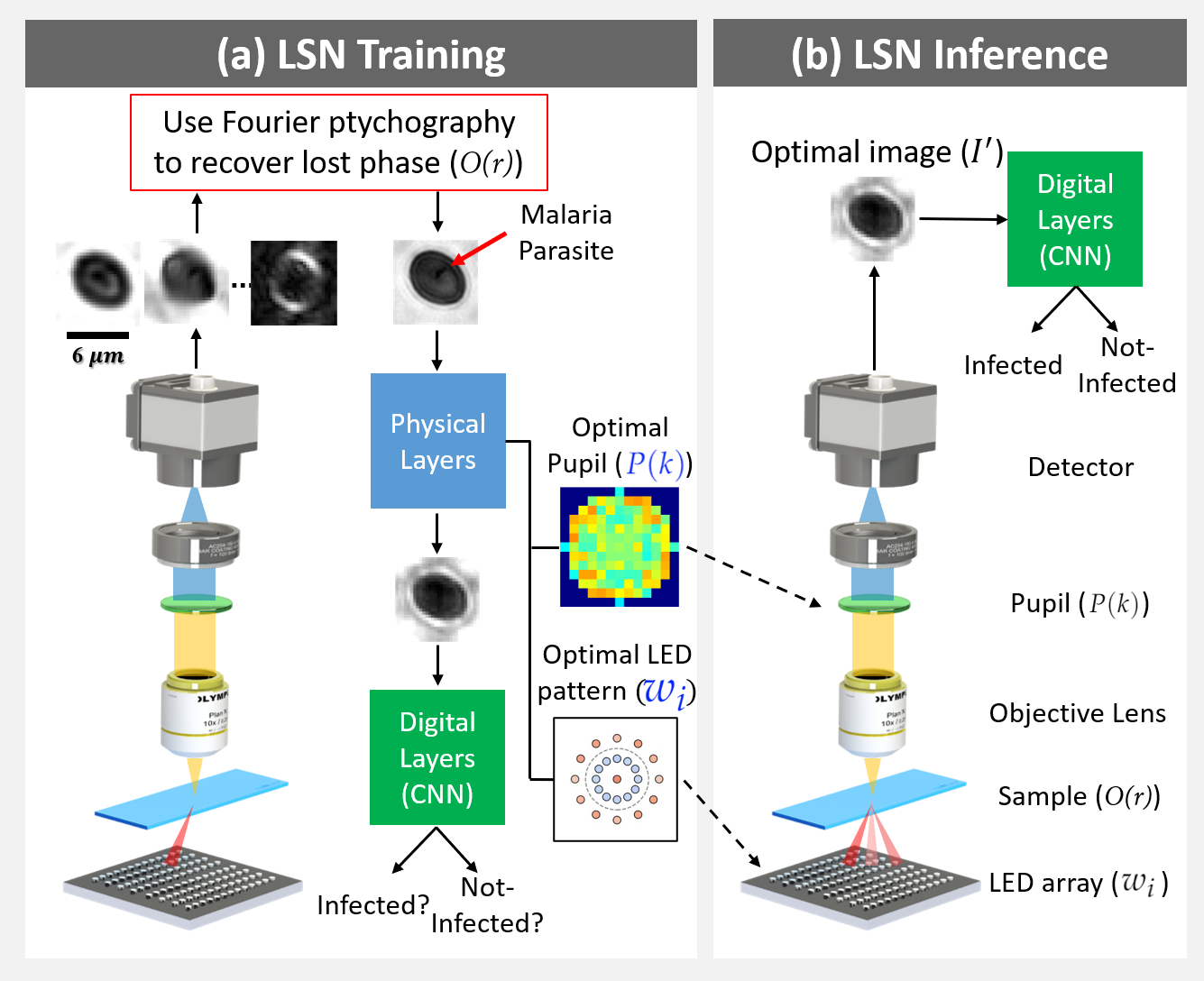
Multi Element microscope optimization
In this work, we investigate an approach to jointly optimize multiple microscope settings, together with a classification network, for improved performance with such automated tasks.
Optics Letters (2020)
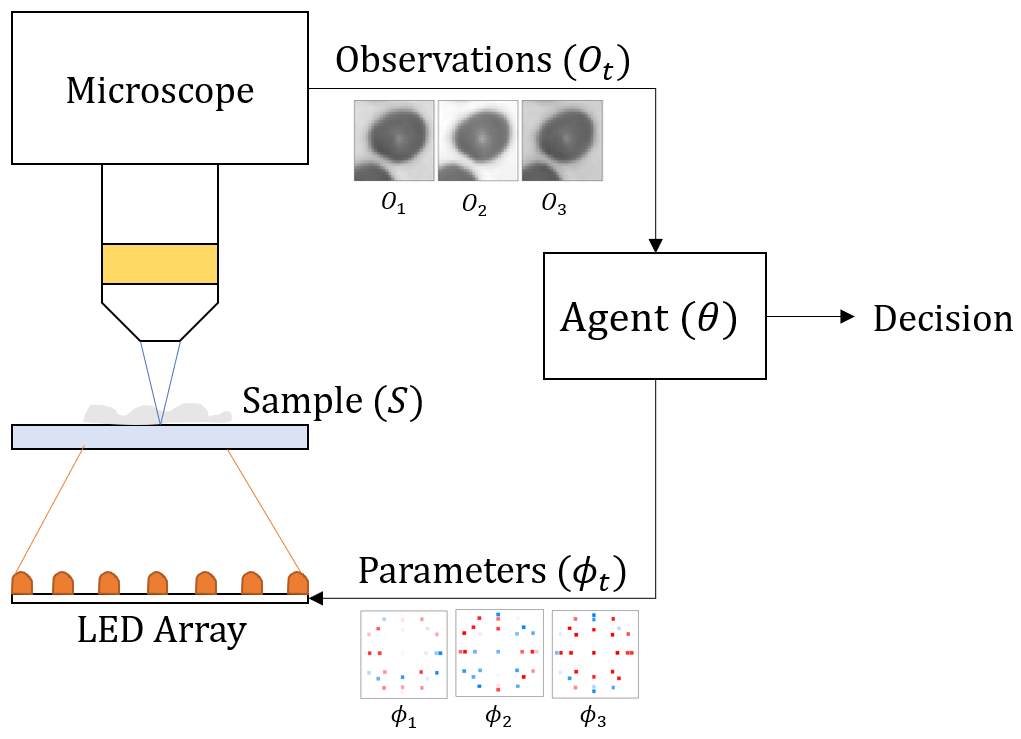
Towards an intelligent microscope
Illumination within a microscope can drastically alter information captured by the image sensor. We present a reinforcement learning system that adaptively explores optimal patterns to illuminate specimens for immediate classification.
ICASSP (2020)
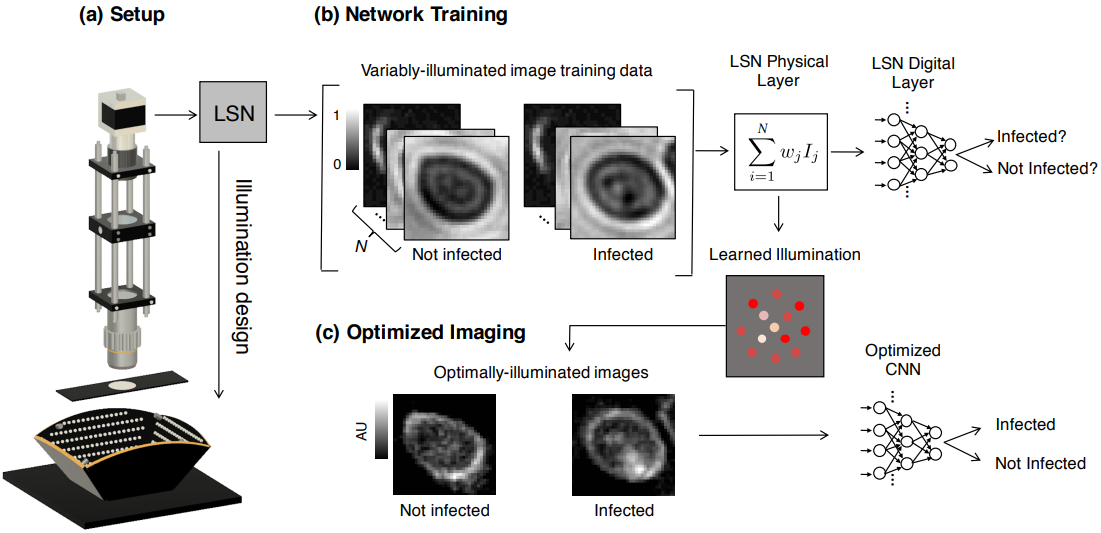
Learned Sensing
To increase the speed and accuracy of automated image classification, this work presents a method to co-optimize how a sample is illuminated in a microscope, along with a pipeline to automatically classify the resulting image, using a deep neural network
Biomedical Optics Express (2019)
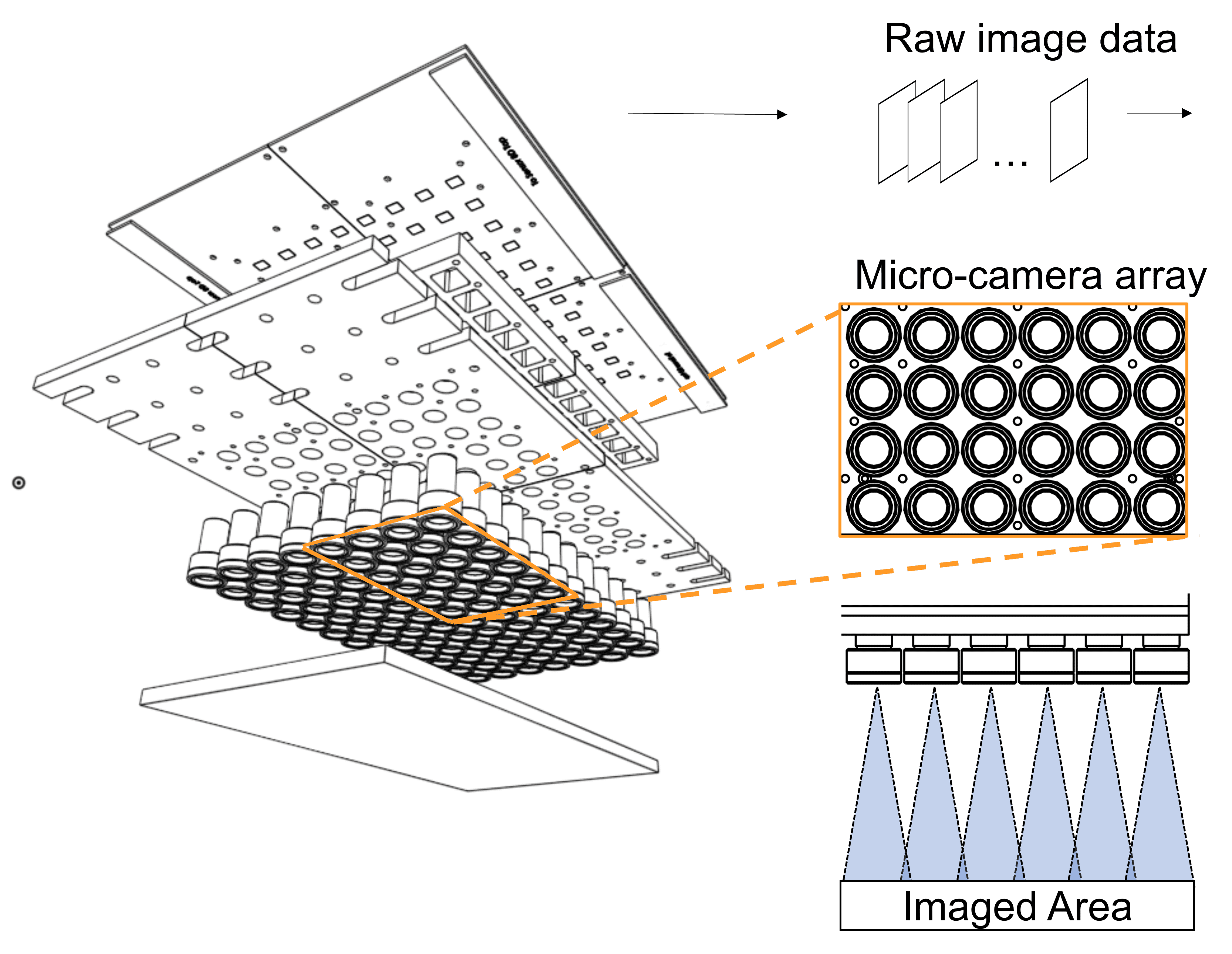
MCAM: The Gigapixel Microscope
We present a novel microscopy approach: Multi Camera Array Microscopy (MCAM), for rapid acquisition of Gigapixel Videos and Images. Also capture 3D Depth measurement, and analyse large FOV sample such as 48 well plates and live freely moving model organisms, with inbuilt algorithms
In preparation…